The Importance of Labeled Image Datasets in Modern Business
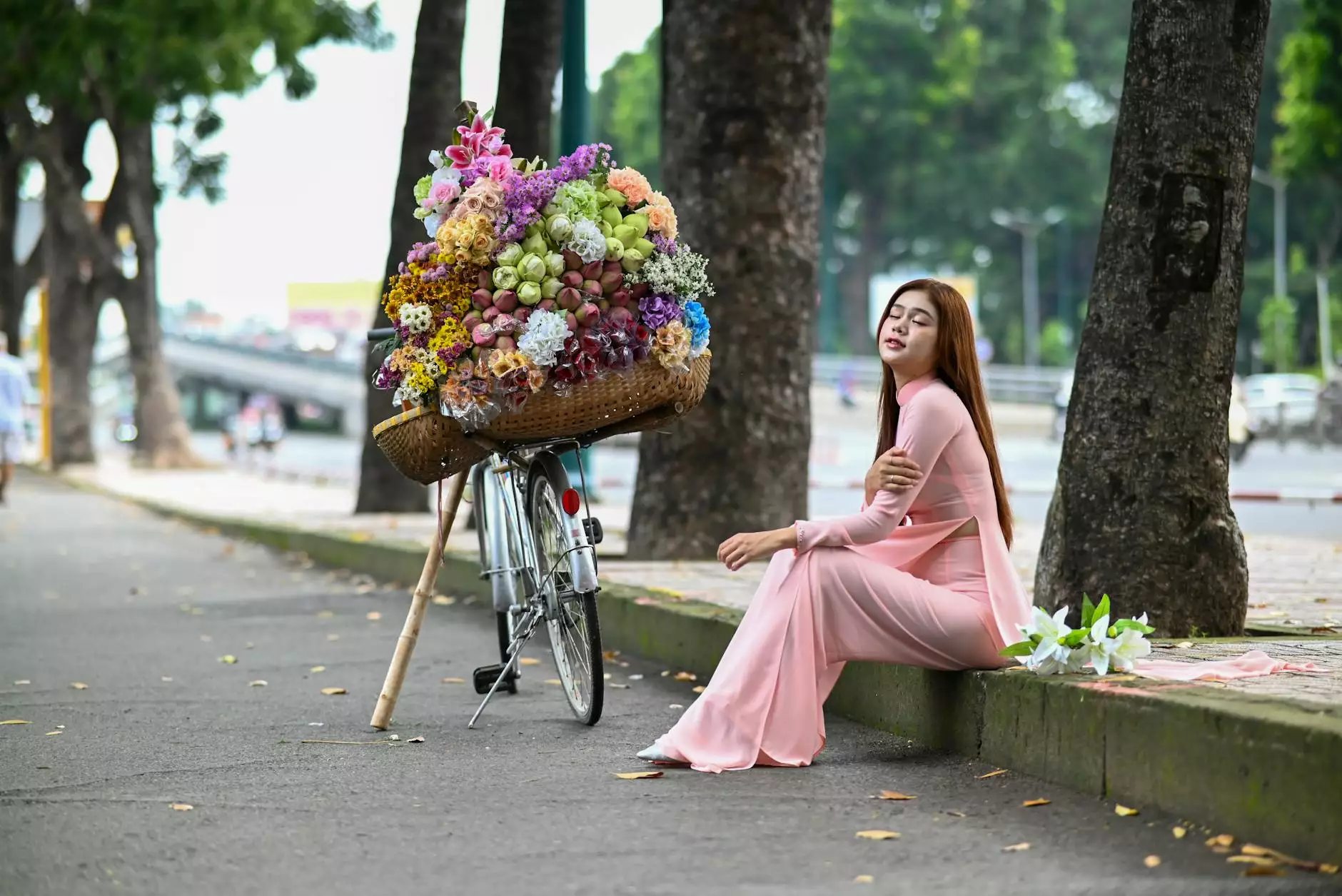
In today’s data-driven world, the significance of labeled image datasets cannot be overstated. Businesses across various sectors are increasingly recognizing the value of these datasets in improving their operations, understanding their customers, and leveraging artificial intelligence (AI) for better decision-making. This article will delve deep into the realm of labeled image datasets, discussing their importance, uses, and how platforms like keylabs.ai are paving the way for businesses to harness their power.
What are Labeled Image Datasets?
Labeled image datasets refer to collections of images that have been annotated with relevant tags or labels. These labels provide critical information about the images, such as identifying objects, actions, or context within the images. The process of labeling images can be time-consuming and requires precision, but the output is invaluable for training machine learning models.
Why Are Labeled Image Datasets Essential?
In the realm of AI and machine learning, the phrase "garbage in, garbage out" holds true. The quality of the data fed into machine learning algorithms directly affects the outcomes. Labeled image datasets are essential for several reasons:
- Training Machine Learning Models: AI models, particularly in computer vision, learn from examples. Labeled datasets provide the necessary training data to improve accuracy.
- Facilitating Object Recognition: Many applications, from security systems to retail, rely on object detection features powered by labeled datasets.
- Improving User Experiences: Businesses use these datasets to understand user preferences and optimize services accordingly.
- Fostering Innovation: With quality data, businesses can create innovative solutions and services that respond better to market demands.
The Role of Data Annotation Tools and Platforms
The process of creating labeled image datasets can be significantly enhanced through the use of advanced data annotation tools and platforms. These tools not only streamline the annotation process but also ensure higher levels of accuracy. Here’s how data annotation platforms contribute to the creation and maintenance of labeled datasets:
1. Automation of Annotation Tasks
Modern data annotation platforms, such as keylabs.ai, utilize AI to assist in the annotation process. Machine learning algorithms can pre-label images, significantly speeding up the process. Human annotators can then verify and refine these labels, ensuring both efficiency and quality. This combination of AI and human oversight maximizes the effectiveness of data annotation tasks.
2. Collaborative Work Environment
Data annotation platforms facilitate team collaboration by allowing multiple users to work on the same project. This collaborative nature ensures that large volumes of data can be processed quickly, and diverse perspectives can enhance the labeling accuracy.
3. Quality Control Mechanisms
Ensuring the quality of labeled datasets is crucial. Top-tier data annotation services implement stringent quality control measures. By employing random sampling and double-checking annotations, they ensure that the labeled image datasets are reliable and useful.
Applications of Labeled Image Datasets
Labeled image datasets find utility across a multitude of industries. Let’s explore some specific applications:
1. Retail and E-commerce
In retail, understanding customer behavior can significantly influence sales. Labeled image datasets can be used to analyze customer interactions with products. For instance, object recognition can help businesses determine which products customers are most interested in by analyzing the images captured in-store or through online platforms.
2. Healthcare
The healthcare industry relies on image analysis for diagnostics. Labeled datasets of medical images, such as X-rays or MRIs, assist AI models in recognizing patterns related to various medical conditions. This not only speeds up diagnosis but also aids in creating new treatments and protocols based on the data.
3. Autonomous Vehicles
Autonomous vehicles depend heavily on accurate image recognition systems. Labeled image datasets are used to train models to understand traffic signs, detect pedestrians, and identify road conditions. This data is vital for developing the technology to ensure the safety and effectiveness of self-driving vehicles.
4. Agriculture
In agriculture, image datasets are utilized for monitoring crop health using drone imagery. By labeling images that indicate healthy versus unhealthy plants, farmers can make better decisions about crop management and disease prevention.